The impact of AI can be seen everywhere in digital marketing, but one place you might not hear as much about is the role of AI in UX. But if you poke around the right corners of LinkedIn and YouTube, you’ll find plenty of examples of people celebrating the ways that AI makes user research, analysis and subsequent design more efficient and data-driven.
At Electric Kite we’re testing and integrating AI tools into various parts of our processes, and as the head of UX I’ve been especially interested to see how we can use AI to improve our work, perhaps not in quality but hopefully as a way to improve efficiency. They claim that AI can automate tedious tasks like survey analysis, wireframing, content suggestions, or A/B testing, so we’re all up for it.
Maybe then I can spend more time writing fun articles about my hobbies and how they relate to work 🙂
Here’s what I’ve learned so far about the good, the bad and the ‘this will probably be better in six months’ using AI in UX research right now. Probably this article will be quite funny in 6 months when I’m expertly using a lot of AI tools and I can laugh about my feeble start with this new technology.
Using AI for Project Immersion and Preparation
Over the last year I’ve asked ChatGPT several times for AI suggestions for creating stakeholder interview questions and user surveys and it has saved me considerable amounts of time, often on repetitive 5-point Likert scales for rating and checking proper grammar for questions.
Crafting the right prompts requires that I use precise wording that explains the project goals and hypothesis or findings we want to get from our target audiences — which also requires a deep understanding of these target audiences. Getting those clear understandings of both the client and project goals as well as a deep understanding of our audience is not always easy to define, and usually requires a lot of time and effort through discussions with our clients and our internal project teams.
The Takeaway: Because those discussions are, by nature, conversations between people, I’d say around 25% of the time it is easier for me to understand the questions that I need to ask in order to define the project and therefore write those scripts myself than asking AI to come up with suggestions.
Using AI for Data Analysis and Summarizing Key Findings
The speed for analyzing large amounts of data is often mentioned as one of the key benefits of AI, and I’ve had mixed experiences with it so far. I find it works well with some well-integrated plugins like the one in Miro for summarizing post-its (see example below) but it can have very inconsistent results with other tools.
Here is an example of an AI-generated research summary of a proto-persona, using Miro’s own AI functionality:
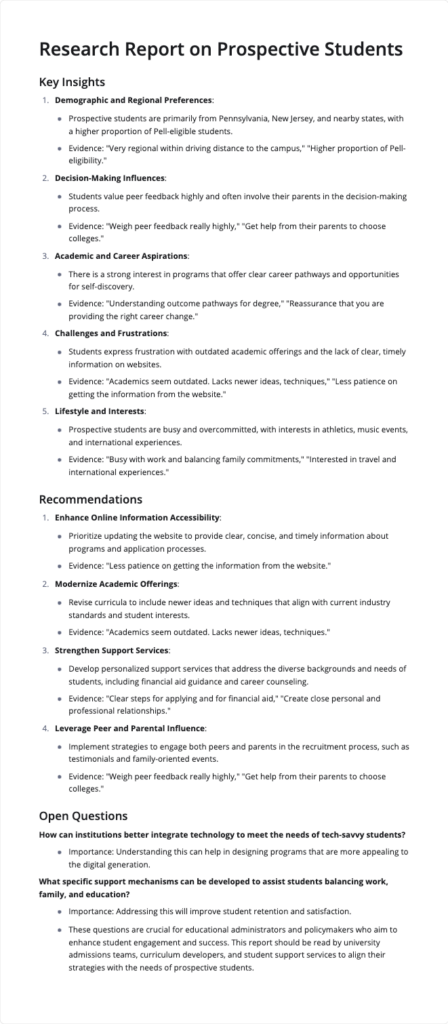
The AI-generated report saved me time by summarizing all the digital post-its from the workshop board and using easy to understand language. It didn’t match the exact structure we use for creating Proto-personas deliverables and I still checked if the information provided was the most accurate. Overall, though, this did save me about 30 minutes, which is definitely nice.
But regarding data analysis, recently I asked ChatGPT to summarize the findings for a survey analysis report and it worked for a set of responses and the next day with a different set but the exact same format, it was giving me formatting errors and not enough data for providing answers.
The Takeaway: Again, the data summaries can be as helpful as the type of questions you follow up with when they provide the analysis. Sometimes the biggest benefit is that it will provide you with the sentence that defines a graph instead of the researcher having to think about it.
Using AI for Wireframe Design
I’ll confess this is the one that I was the most excited about. I expect that all designers feel they can always do better with their designs, so I hoped using AI for wireframe design would be a way of unleashing creativity.
But I’m having mixed results so far. First, on a college website redesign project, we tried asking ChatGPT for some design direction for a university employee dashboard page and we got quite cool content outlines, something that could be easily converted into wireframes for less-experience designers.
Then we asked for a wireframe based on those outlines, and that’s when the disappointment really arrived. Just see it with your own eyes:
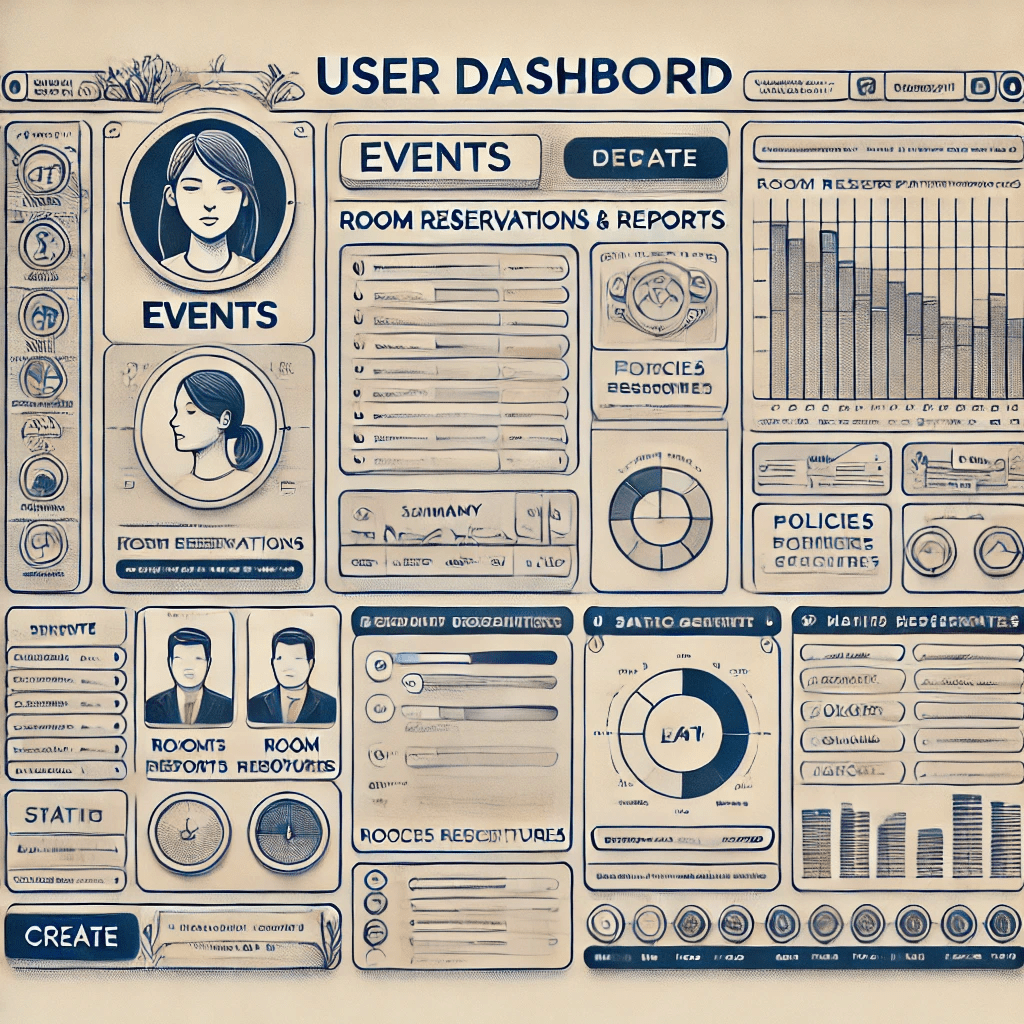
You can probably tell that, while aesthetically pleasing at first glance, if this were actually the dashboard section of a website that managed events and room reservations, it would be a bit of a nightmare to navigate or to even scan for the key information. The components suggested do not meet current digital standards or have any similarity with any style library out there. Not to mention that this looks more like the classified section of a newspaper than an event listing and room reservation management on a modern website.
Even after asking for something cleaner and simplified we got this:
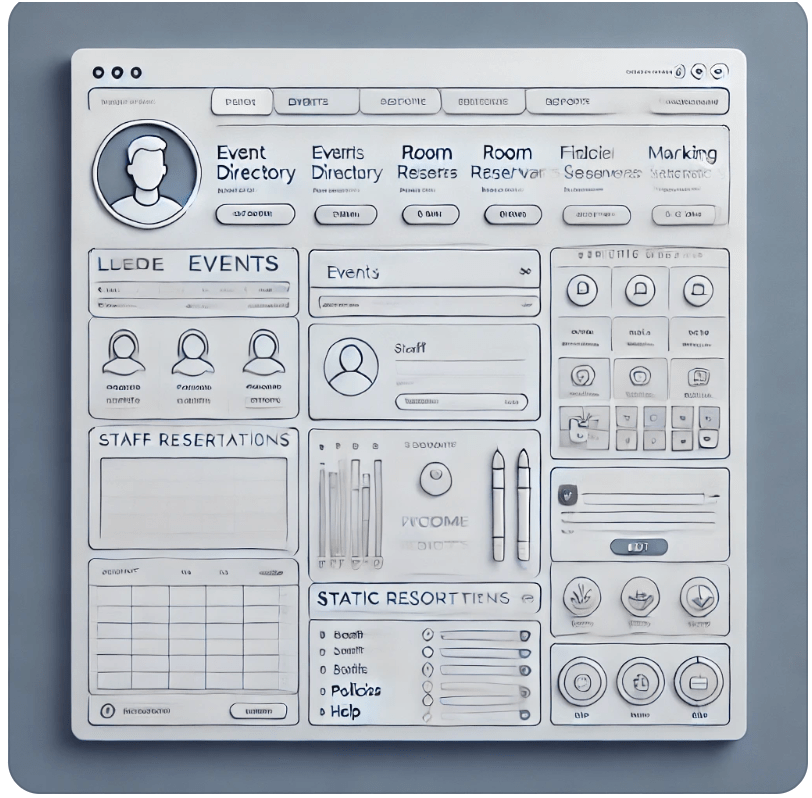
This is only a slight improvement over what was a chaotic mess, above; it is still disorganized, cluttered and generally useless, with little information architecture and clear design for digital components.
The Takeaway: So much for ChatGPT as a tool for initial wireframe ideation. We’re now in the process of trying one of many Figma’s AI tools, and although we’re getting better results, it’s still hard to say that we’re actually saving time and effort.
Using AI for Predictive Analytics with Heuristic Analysis
We’ve been told that AI can forecast user behavior based on past data, helping designers anticipate how users might interact with a website or app. For example, predictive models can inform designers about potential friction points in a user’s journey, allowing proactive design improvements — which is pretty similar to running a heuristic analysis, which is an essential part of every website redesign or development project that we do.
We’ve tried that a few times using ChatGPT and it hasn’t been the best experience. Even providing detailed prompts with specific context around project goals, intended uses of the website and our detailed findings from our research, the findings and recommendations do not match the expectations that we had.
The Takeaway: While we haven’t found AI as a useful tool for predictive analysis related to heuristics, one way that AI has helped us in this area so far as been by creating succinct, easy-to-understand explanation for clients about why, for example, their website should not use various features such as image carousels, red font-color or a right hand side navigation, instead of using its findings and recommendations. Because the one thing we know AI can do right now is generate expert-condensing language into readable text (that we then review and edit as necessary by handing off, of course).
What AI Can’t Do in UX: Emotion and Empathy
AI tools, while powerful, cannot fully replicate human cognition, empathy, or the nuanced understanding of individual user preferences. They cannot really tell if the user’s hesitation to respond to a simple question is because they truly are confused or because they are not paying attention to the test. AI cannot pick up sarcasm or irony, cannot really (naturally) joke with a user to set them at ease, and definitely cannot put themselves in the shoes of the user.
Human researchers excel at asking why without making the user feel uncomfortable, they know how to interpret if the user is not focused or how to react when disruptions happen during a test.
Real user testing is necessary to identify gaps, emotional responses, and unexpected behaviors that AI might overlook. Having researchers, designers, developers and all types of project stakeholders listen, observe and even interact with users is the key to developing the empathy needed to fully, deeply understand the experience of target users when interacting with our products, services and websites. And funnily enough, to understand those pesky project goals and website success criteria that will help us use AI tools to its fullest potential.
Why We Always Use Humans to Review AI Recommendations
And while AI has made significant progress in generating recommendations and suggestions for those laborious tasks, we still need human experts to review these outputs because the output of these AI tools are as reliable as we’d like (yet).
AI-generated suggestions often focus on optimizing quantitative metrics like clicks or engagement but often miss subtleties and qualitative outcomes like the emotional resonance of a design or its accessibility for diverse user groups. UX researchers and designers are key in ensuring that AI recommendations are aligned with design principles and ethical considerations, creating experiences that truly resonate with users.
Furthermore, UX and interaction design require an understanding of context, which we’ve already mentioned that AI doesn’t fully grasp. For instance, AI might suggest design changes based on past trends or current data, but a human expert can evaluate whether these changes fit the brand, the product’s goals, or the unique needs of the target audience and sometimes even if they actually are doable, make sense and are not contradictory or disruptive with other recommendations. Human experts also bring creative insight to the process—something AI struggles to replicate. By reviewing the suggestions provided by AI, designers can combine the efficiency and data-driven insights with human creativity, empathy, and intuition, ensuring that the final product not only performs well but also connects meaningfully with users.
The Big Takeaway for AI in UX
AI can be a valuable tool in UX design, enhancing efficiency and data processing capabilities, but the human element remains irreplaceable for truly intuitive and emotionally resonant designs as well as reviewing and pretty much hand-holding the tools while delivering the quality of results our projects deserve.
We still need humans to be human and create human experiences. We still need to humanize technology, even (especially?) when it’s as wildly popular and being adopted as rapidly as AI.
Want to talk about how we can help your organization through UX research? Let’s talk.